Annual Report 2020
Division of Molecular Modification and Cancer Biology
Ryuji Hamamoto, Syuzo Kaneko, Masaaki Komatsu, Ken Asada, Satoshi Takahashi, Ken Takasawa, Masayoshi Yamada, Masamichi Takahashi, Kazuma Kobayashi, Kyoko Fujioka, Noriko Ikawa, Hiroko Kondo, Shigemi Yamada, Hidenori Machino, Amina Bolatkan, Norio Shinkai, Kanto Shozu, Ai Dozen, Ryo Shimoyama, Akira Sakai
Introduction
This division has been functioning as the core of medical AI research and development at the Tsukiji Campus since 2016, when a project to develop an integrated cancer medical system has been started using artificial intelligence (AI) technology as part of the CREST project of the Japan Science and Technology Agency (JST). In 2018, the project was added on to the CREST project of the Cabinet Office's Public-Private R&D Investment Program (PRISM) "Development of Artificial Intelligence to Accelerate the Creation of New Drugs", and we are promoting research in close collaboration with staffs from 17 departments and divisions of the Central Hospital. In particular, the following two points have been emphasized in our research activities: 1) Aiming for actual clinical application: promoting research for patients without falling into research for research's sake, and 2) Building an integrated database that includes a wealth of high-quality medical information.
Research activities
1. Development of RCRA ChIP-seq Analysis Method for Application to Precision Medicine
We have developed the RCRA ChIP-seq method as a basis for multilayer omics analysis, and succeeded in developing a protocol for efficient ChIP-seq analysis from FFPE tissues. By using this method, identifying the binding sites of transcription factors ahead of others in the world has been successful. In this system, a robot was also introduced to enable highly reproducible and accurate data acquisition, and the results have already been published as an original paper in a peer-reviewed international journal.
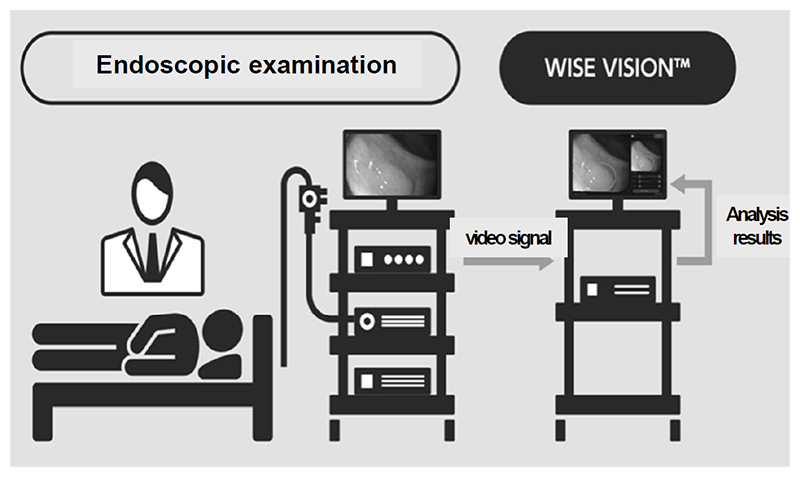
Figure 2. Example of colorectal cancer detection using the endoscopic AI system.
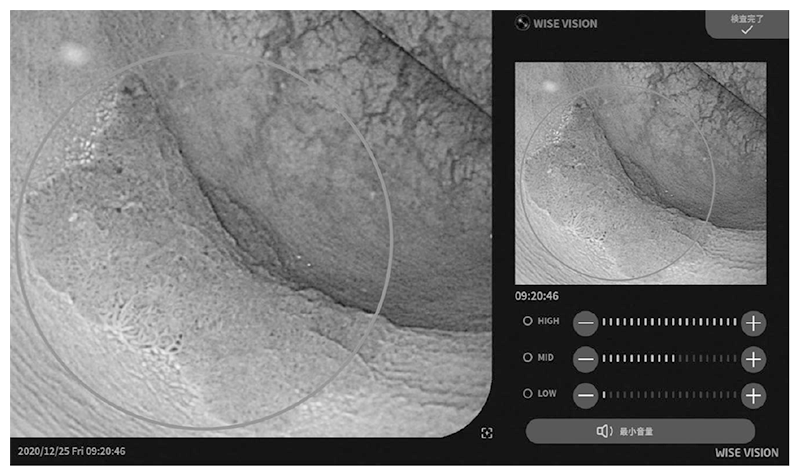
2. Development of medical image diagnosis system using AI technology
The real-time endoscopic diagnostic support system using AI was approved by the PMDA as a controlled medical device (class II) (approval number: 30200BZX00382000), and has also complied with the requirements for the CE mark, which is a standard for medical device products in Europe (Fig. 1 and Fig. 2). With the approval of the medical device, our research results are now being used in clinical practice in Japan and Europe, and with the support of AI, lesions can be detected with less influence of the endoscopist's experience, and with fewer false positives, the diagnostic accuracy is expected to be improved without increasing the examination time.
3. Construction of the world's largest integrated lung cancer database and development of a multi-omics analysis platform using AI technology
We are constructing the world's largest lung cancer integrated database with more than 1600 cases. Moreover, we have constructed a system to efficiently collect medical information and medical images of various modalities from electronic medical records and pathology systems in conjunction with the construction of the integrated database. In addition, we have developed several types of platforms using AI technology to efficiently and precisely analyze large-scale omics data related to cancer.
Education
A total of six graduate students from Tokyo Medical and Dental University, Juntendo University, Toyama University, and Keio University belonged to our department and provided research guidance. We also actively participated in young research seminars organized by the Research Institute, and a member of our group received the Director's Award.
Future Prospects
1. Using the RCRA ChIP-seq method, we will conduct ChIP-seq analysis using large-scale lung cancer clinical specimens, and integrate the analysis with other omics analysis including whole genome analysis and RNA-seq analysis.
2. As for the endoscopic AI system, we are aiming for medical device approval for qualitative diagnosis. We will also aim for the clinical application of other AI-based medical image analysis including radiology image analysis.
3. In addition to expanding the lung cancer integrated database, we will continue to develop an omics analysis platform using machine learning and deep learning technologies.
List of papers published in 2020
Journal
1. Takayanagi D, Hirose S, Kuno I, Asami Y, Murakami N, Matsuda M, Shimada Y, Sunami K, Komatsu M, Hamamoto R, Kato MK, Matsumoto K, Kohno T, Kato T, Shiraishi K, Yoshida H. Comparative Analysis of Genetic Alterations, HPV-Status, and PD-L1 Expression in Neuroendocrine Carcinomas of the Cervix. Cancers (Basel), 13:2021
2. Takahashi S, Takahashi M, Kinoshita M, Miyake M, Kawaguchi R, Shinojima N, Mukasa A, Saito K, Nagane M, Otani R, Higuchi F, Tanaka S, Hata N, Tamura K, Tateishi K, Nishikawa R, Arita H, Nonaka M, Uda T, Fukai J, Okita Y, Tsuyuguchi N, Kanemura Y, Kobayashi K, Sese J, Ichimura K, Narita Y, Hamamoto R. Fine-Tuning Approach for Segmentation of Gliomas in Brain Magnetic Resonance Images with a Machine Learning Method to Normalize Image Differences among Facilities. Cancers (Basel), 13:2021
3. Ozawa T, Kaneko S, Szulzewsky F, Qiao Z, Takadera M, Narita Y, Kondo T, Holland EC, Hamamoto R, Ichimura K. C11orf95-RELA fusion drives aberrant gene expression through the unique epigenetic regulation for ependymoma formation. Acta Neuropathol Commun, 9:36, 2021
4. Kageyama SI, Du J, Kaneko S, Hamamoto R, Yamaguchi S, Yamashita R, Okumura M, Motegi A, Hojo H, Nakamura M, Tsuchihara K, Akimoto T. Identification of the mutation signature of the cancer genome caused by irradiation. Radiother Oncol, 155:10-16, 2021
5. Kobayashi K, Murakami N, Takahashi K, Inaba K, Igaki H, Hamamoto R, Itami J. Tensor Regression-based Model to Investigate Heterogeneous Spatial Radiosensitivity After I-125 Seed Implantation for Prostate Cancer. In Vivo, 35:489-497, 2021
6. Hamamoto R. Application of Artificial Intelligence for Medical Research. Biomolecules, 11:2021
7. Komatsu M, Sakai A, Komatsu R, Matsuoka R, Yasutomi S, Shozu K, Dozen A, Machino H, Hidaka H, Arakaki T, Asada K, Kaneko S, Sekizawa A, Hamamoto R. Cardiac Structural Abnormalities in Fetal Ultrasound Videos Using Deep Learning. Applied Sciences, 11:371, 2021
8. Yasutomi S, Arakaki T, Matsuoka R, Sakai A, Komatsu R, Shozu K, Dozen A, Machino H, Asada K, Kaneko S, Dozen A, Machino H, Asada K, Kaneko S, Sekizawa A, Hamamoto R, Komatsu M. Shadow Estimation for Ultrasound Images Using Auto-Encoding Structures and Synthetic Shadows. Applied Sciences, 11:1127, 2021
9. Saloura V, Vougiouklakis T, Bao R, Kim S, Baek S, Zewde M, Bernard B, Burkitt K, Nigam N, Izumchenko E, Dohmae N, Hamamoto R, Nakamura Y. WHSC1 monomethylates histone H1 and induces stem-cell like features in squamous cell carcinoma of the head and neck. Neoplasia, 22:283-293, 2020
10. Asada K, Bolatkan A, Takasawa K, Komatsu M, Kaneko S, Hamamoto R. Critical Roles of N(6)-Methyladenosine (m(6)A) in Cancer and Virus Infection. Biomolecules, 10:2020
11. Jinnai S, Yamazaki N, Hirano Y, Sugawara Y, Ohe Y, Hamamoto R. The Development of a Skin Cancer Classification System for Pigmented Skin Lesions Using Deep Learning. Biomolecules, 10:2020
12. Kobayashi K, Bolatkan A, Shiina S, Hamamoto R. Fully-Connected Neural Networks with Reduced Parameterization for Predicting Histological Types of Lung Cancer from Somatic Mutations. Biomolecules, 10:2020
13. Kojima M, Sone K, Oda K, Hamamoto R, Kaneko S, Oki S, Kukita A, Kawata A, Honjoh H, Kawata Y, Kashiyama T, Sato M, Taguchi A, Miyamoto Y, Tanikawa M, Tsuruga T, Nagasaka K, Wada-Hiraike O, Osuga Y, Fujii T. The histone methyltransferase SMYD2 is a novel therapeutic target for the induction of apoptosis in ovarian clear cell carcinoma cells. Oncol Lett, 20:153, 2020
14. Takahashi S, Asada K, Takasawa K, Shimoyama R, Sakai A, Bolatkan A, Shinkai N, Kobayashi K, Komatsu M, Kaneko S, Sese J, Hamamoto R. Predicting Deep Learning Based Multi-Omics Parallel Integration Survival Subtypes in Lung Cancer Using Reverse Phase Protein Array Data. Biomolecules, 10:2020
15. Dozen A, Komatsu M, Sakai A, Komatsu R, Shozu K, Machino H, Yasutomi S, Arakaki T, Asada K, Kaneko S, Matsuoka R, Aoki D, Sekizawa A, Hamamoto R. Image Segmentation of the Ventricular Septum in Fetal Cardiac Ultrasound Videos Based on Deep Learning Using Time-Series Information. Biomolecules, 10:2020
16. Papadaki V, Asada K, Watson JK, Tamura T, Leung A, Hopkins J, Dellett M, Sasai N, Davaapil H, Nik-Zainal S, Longbottom R, Nakakido M, Torii R, Veerakumarasivam A, Kaneko S, Sagoo MS, Murphy G, Mitani A, Tsumoto K, Kelly JD, Hamamoto R, Ohnuma SI. Two Secreted Proteoglycans, Activators of Urothelial Cell-Cell Adhesion, Negatively Contribute to Bladder Cancer Initiation and Progression. Cancers (Basel), 12:2020
17. Hamamoto R, Suvarna K, Yamada M, Kobayashi K, Shinkai N, Miyake M, Takahashi M, Jinnai S, Shimoyama R, Sakai A, Takasawa K, Bolatkan A, Shozu K, Dozen A, Machino H, Takahashi S, Asada K, Komatsu M, Sese J, Kaneko S. Application of Artificial Intelligence Technology in Oncology: Towards the Establishment of Precision Medicine. Cancers (Basel), 12:2020
18. Wada M, Kukita A, Sone K, Hamamoto R, Kaneko S, Komatsu M, Takahashi Y, Inoue F, Kojima M, Honjoh H, Taguchi A, Kashiyama T, Miyamoto Y, Tanikawa M, Tsuruga T, Mori-Uchino M, Wada-Hiraike O, Osuga Y, Fujii T. Epigenetic Modifier SETD8 as a Therapeutic Target for High-Grade Serous Ovarian Cancer. Biomolecules, 10:2020
19. Shozu K, Komatsu M, Sakai A, Komatsu R, Dozen A, Machino H, Yasutomi S, Arakaki T, Asada K, Kaneko S, Matsuoka R, Nakashima A, Sekizawa A, Hamamoto R. Model-Agnostic Method for Thoracic Wall Segmentation in Fetal Ultrasound Videos. Biomolecules, 10:2020